集成学习可以分为两大类:
- 学习器之间存在依赖关系,必须串行生成序列化方法。
- 学习器之间不存在依赖关系,可以同时生成的并行化方法。
前者的代表是“Boosting”,后者的代表是“Bagging”和“随机森林(Random Forest)”.
得到泛化能力强的集成,每个学习器要尽量不同,如何做到不同。一个方法是由训练集产生多个不同的子集,在每个子集上训练学习器。如5000个样本,用5个学习器分别学习1000个样本集。如此产生5个不同的模型。但是如此一来,每个模型的性能会有所下降,同时,集成学习的一个优势是每个学习器并不需要具有很强的性能。
从数据中采样
但是,“好而不同”毕竟每个学习器要“好”。所以根据每个学习器只使用数据的一部分,产生两种采样方式:
- 有放回抽样 自助采样(Bootstrap Sampling)
- 无放回抽样
自助采样:假设原始数据集由m个样本,对于第一个学习器,随机采样一个样本,放入采样集中,后把该样本放回原数据集。如此采样m次便得到含有m个样本的子集来训练学习器#1
。对于其他学习器,采用同样的方式得到训练集。如此得到的不同子集中一定存在重复的元素。最后基于每一个子集训练学习器,后集成。此过程成为Bagging
。
对于无放回抽样,就如上述所述,5000个样本是数据集,若分给5个学习器,每个得到1000个样本;10 个学习器,每个得到500个样本。这种方法成为Pasting
。其局限性很显然,学习器个数与其子集样本数成反比。
Bagging常用。例:
所使用数据集:
1 | x, y = datasets.make_moons(n_samples=1000, noise=0.2, random_state=111) |
使用决策树为基学习器,设置5
个,采样为放回采样:
1 | from sklearn.tree import DecisionTreeClassifier |
测试集的正确率为:94.4%
设置500
个基学习器:
1 | bagg2 = BaggingClassifier(DecisionTreeClassifier(), |
测试集准确率: 96.0%
Out-of-bag Estimate(OOB)
可以计算,通过自助采样,原始数据集中大约有36.8%的样本未出现在每个学习器的样本子集中。所以对于Bagging,天然的,在原始数据集中就有测试集了,即那剩下的原始数据集中的36.7%。
使用sklearn 中的oob_score_
实现oob
:
在放回采样过程中,记录那些样本没有被取到,这些未被取到的作为验证集或测试集,这个过程由oob_score=True 实现:
1 | bagg3 = BaggingClassifier(DecisionTreeClassifier(), |
结果0.958。
多核心并行
由于每个学习器没有相互影响,所以所有学习器可以同时学习:
指定n_jobs
的值,当其为-1
时,表示使用计算机所有物理核心:
1 | # 并行执行,使用所有核心 |
计时得到最终执行时间:0.1591450000000001
而使用一个核心的执行时间为:0.6764169999999998
更多采样方式
当数据集的特征较多时,可是对特征进行随机采样:Random Subspaces
。只在列上随机采样。如下左图。
另一种采样方式,既对样本随机采样,又对特征的随机采样:Random Patches
。既在行又在列上随机采样。如下右图:
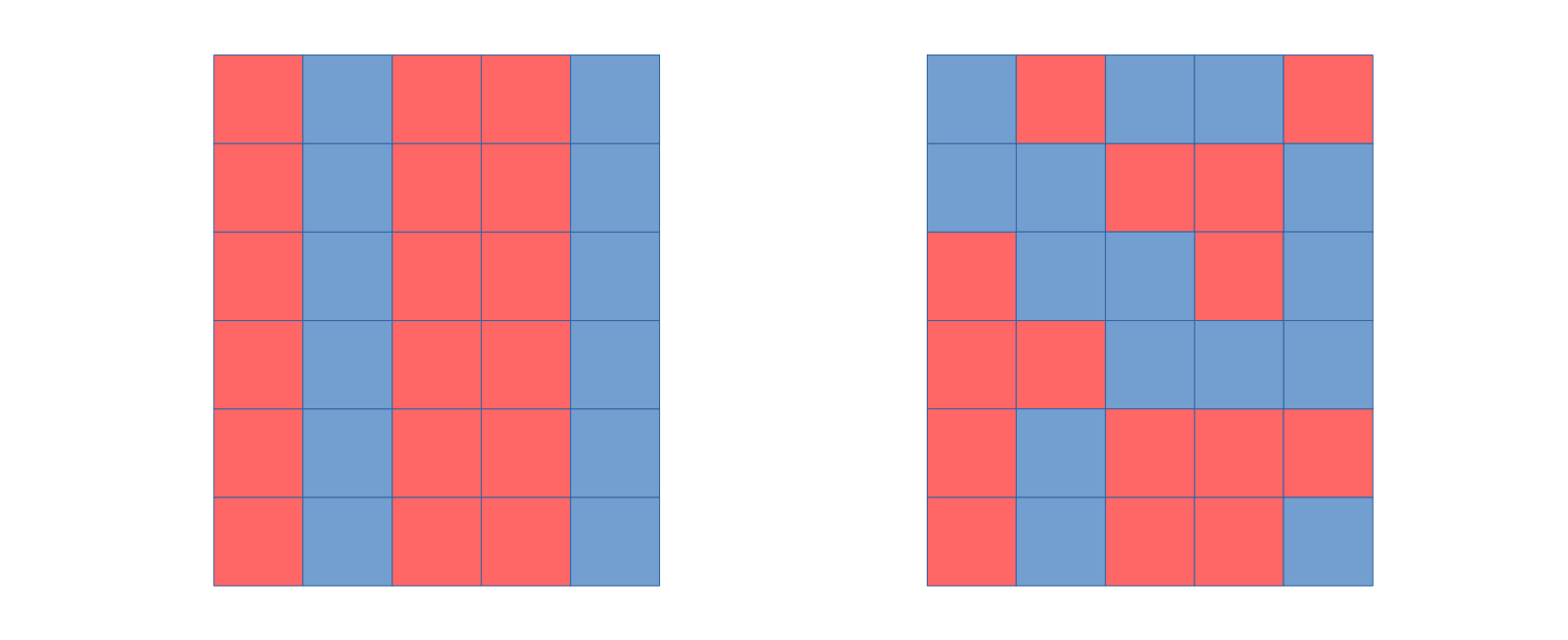
既然有对样本数量的自助采样(bootstrap sampling),也有对样本特征的自助采样。如此得到的特征为bootstrap features
:
Random Subspaces
只对特征进行随机采样,将学习机的
bootstrap_features
参数置为True
:1
2
3
4
5
6
7
8
9
10
11
12
13
14# 关闭样数量的随机采样
random_subspaces_bag = BaggingClassifier(DecisionTreeClassifier(),
n_estimators=500,
max_samples=x.shape[0], # 所有行
max_features=1,
bootstrap=True,
bootstrap_features=True, # 一部分列
oob_score=True,
n_jobs=-1)
start3 = clock()
random_subspaces_bag.fit(x, y)
end3 = clock()
print(random_subspaces_bag.oob_score_)
print(end3 - start3)结果为: 0.835
运行时间:0.1876460000000002
Random Patches,
既对样本数据量采样,又对特征进行采样。因为原始数据只有2个特征,所以此处只采样一个特征。
1
2
3
4
5
6
7
8
9
10
11
12
13
14## random patches 既有样本数的随机采样, 又有特征的随机采样:
random_patches_bag = BaggingClassifier(DecisionTreeClassifier(),
n_estimators=500,
max_samples=200, # 一部分行
max_features=1,
bootstrap=True,
bootstrap_features=True, # 一部分列
oob_score=True,
n_jobs=-1)
start3 = clock()
random_patches_bag.fit(x, y)
end3 = clock()
print(random_patches_bag.oob_score_)
print(end3 - start3)结果为:0.897
运行时间: 0.16168400000000016对于图像信息,除了pooling采样操作,还可以使用这两种采样操作。
敲黑板
”好而不同“是集成学习的核心。
”不同“的实现方式之一是”采样“:对样本数量采样,对样本特征采样。
即,使用一部分数据训练基学习器。